Top 65+ Data Analyst Interview Questions and Answers [2024] – Simplilearn
Lesson 8 of 10By Shruti M
Data analytics is widely used in every sector in the 21st century. A career in the field of data analytics is highly lucrative in today's times, with its career potential increasing by the day. Out of the many job roles in this field, a data analyst's job role is widely popular globally. A data analyst collects and processes data; he/she analyzes large datasets to derive meaningful insights from raw data.
If you have plans to apply for a data analyst's post, then there are a set of data analyst interview questions that you have to be prepared for. In this article, you will be acquainted with the top data analyst interview questions, which will guide you in your interview process. So, let’s start with our generic data analyst interview questions.
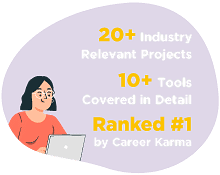
In an interview, these questions are more likely to appear early in the process and cover data analysis at a high level.
Data Wrangling is the process wherein raw data is cleaned, structured, and enriched into a desired usable format for better decision making. It involves discovering, structuring, cleaning, enriching, validating, and analyzing data. This process can turn and map out large amounts of data extracted from various sources into a more useful format. Techniques such as merging, grouping, concatenating, joining, and sorting are used to analyze the data. Thereafter it gets ready to be used with another dataset.
This is one of the most basic data analyst interview questions. The various steps involved in any common analytics projects are as follows:
Understand the business problem, define the organizational goals, and plan for a lucrative solution.
Gather the right data from various sources and other information based on your priorities.
Clean the data to remove unwanted, redundant, and missing values, and make it ready for analysis.
Use data visualization and business intelligence tools, data mining techniques, and predictive modeling to analyze data.
Interpret the results to find out hidden patterns, future trends, and gain insights.
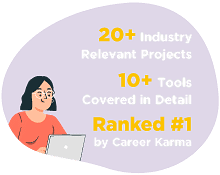
The common problems steps involved in any analytics project are:
As a data analyst, you are expected to know the tools mentioned below for analysis and presentation purposes. Some of the popular tools you should know are:
For working with data stored in relational databases
For creating reports and dashboards
For statistical analysis, data modeling, and exploratory analysis
For presentation, displaying the final results and important conclusions
It provides insights into the past to answer “what has happened”
Understands the future to answer “what could happen”
Suggest various courses of action to answer “what should you do”
Uses data aggregation and data mining techniques
Uses statistical models and forecasting techniques
Uses simulation algorithms and optimization techniques to advise possible outcomes
Example: An ice cream company can analyze how much ice cream was sold, which flavors were sold, and whether more or less ice cream was sold than the day before
Example: An ice cream company can analyze how much ice cream was sold, which flavors were sold, and whether more or less ice cream was sold than the day before
Example: Lower prices to increase the sale of ice creams, produce more/fewer quantities of a specific flavor of ice cream
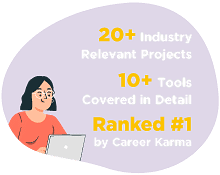
Sampling is a statistical method to select a subset of data from an entire dataset (population) to estimate the characteristics of the whole population.
There are majorly five types of sampling methods:
Univariate analysis is the simplest and easiest form of data analysis where the data being analyzed contains only one variable.
Example - Studying the heights of players in the NBA.
Univariate analysis can be described using Central Tendency, Dispersion, Quartiles, Bar charts, Histograms, Pie charts, and Frequency distribution tables.
The bivariate analysis involves the analysis of two variables to find causes, relationships, and correlations between the variables.
Example – Analyzing the sale of ice creams based on the temperature outside.
The bivariate analysis can be explained using Correlation coefficients, Linear regression, Logistic regression, Scatter plots, and Box plots.
The multivariate analysis involves the analysis of three or more variables to understand the relationship of each variable with the other variables.
Example – Analysing Revenue based on expenditure.
Multivariate analysis can be performed using Multiple regression, Factor analysis, Classification & regression trees, Cluster analysis, Principal component analysis, Dual-axis charts, etc.
The answer to this question may vary from a case to case basis. However, some general strengths of a data analyst may include strong analytical skills, attention to detail, proficiency in data manipulation and visualization, and the ability to derive insights from complex datasets. Weaknesses could include limited domain knowledge, lack of experience with certain data analysis tools or techniques, or challenges in effectively communicating technical findings to non-technical stakeholders.
Some of the most the ethical considerations of data analysis includes:
You should name the tools you have used personally, however here’s a list of the commonly used data visualization tools in the industry:
This is one of the most frequently asked data analyst interview questions, and the interviewer expects you to give a detailed answer here, and not just the name of the methods. There are four methods to handle missing values in a dataset.
In the listwise deletion method, an entire record is excluded from analysis if any single value is missing.
Take the average value of the other participants' responses and fill in the missing value.
You can use multiple-regression analyses to estimate a missing value.
It creates plausible values based on the correlations for the missing data and then averages the simulated datasets by incorporating random errors in your predictions.
Normal Distribution refers to a continuous probability distribution that is symmetric about the mean. In a graph, normal distribution will appear as a bell curve.

Time Series analysis is a statistical procedure that deals with the ordered sequence of values of a variable at equally spaced time intervals. Time series data are collected at adjacent periods. So, there is a correlation between the observations. This feature distinguishes time-series data from cross-sectional data.
Below is an example of time-series data on coronavirus cases and its graph.

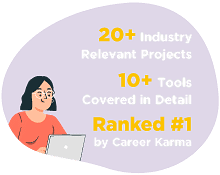
This is another frequently asked data analyst interview question, and you are expected to cover all the given differences!
Happens when the model learns the random fluctuations and noise in the training dataset in detail.
This happens when there is lesser data to build an accurate model and when we try to develop a linear model using non-linear data.

An outlier is a data point that is distant from other similar points. They may be due to variability in the measurement or may indicate experimental errors.
The graph depicted below shows there are three outliers in the dataset.

To deal with outliers, you can use the following four methods:
Hypothesis testing is the procedure used by statisticians and scientists to accept or reject statistical hypotheses. There are mainly two types of hypothesis testing:
Example: There is no association between a patient’s BMI and diabetes.
Example: There could be an association between a patient’s BMI and diabetes.
In Hypothesis testing, a Type I error occurs when the null hypothesis is rejected even if it is true. It is also known as a false positive.
A Type II error occurs when the null hypothesis is not rejected, even if it is false. It is also known as a false negative.
Ans: The choice of handling technique depends on factors such as the amount and nature of missing data, the underlying analysis, and the assumptions made. It's crucial to exercise caution and carefully consider the implications of the chosen approach to ensure the integrity and reliability of the data analysis. However, a few solutions could be:
Outlier detection is the process of identifying observations or data points that significantly deviate from the expected or normal behavior of a dataset. Outliers can be valuable sources of information or indications of anomalies, errors, or rare events.
It's important to note that outlier detection is not a definitive process, and the identified outliers should be further investigated to determine their validity and potential impact on the analysis or model. Outliers can be due to various reasons, including data entry errors, measurement errors, or genuinely anomalous observations, and each case requires careful consideration and interpretation.

Future-Proof Your AI/ML Career: Top Dos and Don'ts
Free Webinar | 5 Dec, Tuesday | 7 PM ISTRegister Now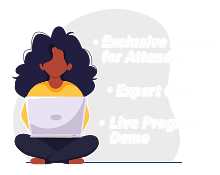
Yes, you can provide a dynamic range in the “Data Source” of Pivot tables. To do that, you need to create a named range using the offset function and base the pivot table using a named range constructed in the first step.
The get the day of the week, you can use the WEEKDAY() function.
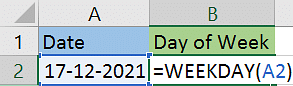
The above function will return 6 as the result, i.e., 17th December is a Saturday.
AND() is a logical function that checks multiple conditions and returns TRUE or FALSE based on whether the conditions are met.
Syntax: AND(logica1,[logical2],[logical3]....)
In the below example, we are checking if the marks are greater than 45. The result will be true if the mark is >45, else it will be false.
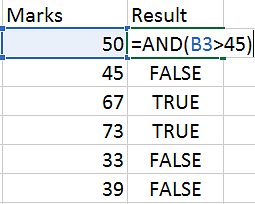
VLOOKUP is used when you need to find things in a table or a range by row.
VLOOKUP accepts the following four parameters:
lookup_value - The value to look for in the first column of a table
table - The table from where you can extract value
col_index - The column from which to extract value
range_lookup - [optional] TRUE = approximate match (default). FALSE = exact match
Let’s understand VLOOKUP with an example.

If you wanted to find the department to which Stuart belongs to, you could use the VLOOKUP function as shown below:

Here, A11 cell has the lookup value, A2:E7 is the table array, 3 is the column index number with information about departments, and 0 is the range lookup.
If you hit enter, it will return “Marketing”, indicating that Stuart is from the marketing department.
In Excel, you can use the TODAY() and NOW() function to get the current date and time.


You can use the SUMIFS() function to find the total quantity.
For the Sales Rep column, you need to give the criteria as “A*” - meaning the name should start with the letter “A”. For the Cost each column, the criteria should be “>10” - meaning the cost of each item is greater than 10.

The result is 13.






To subset or filter data in SQL, we use WHERE and HAVING clauses.
Consider the following movie table.

Using this table, let’s find the records for movies that were directed by Brad Bird.

Now, let’s filter the table for directors whose movies have an average duration greater than 115 minutes.

Answer all of the given differences when this data analyst interview question is asked, and also give out the syntax for each to prove your thorough knowledge to the interviewer.
HAVING is used to filter values from a group.
Syntax of WHERE clause:
SELECT column1, column2, ...
FROM table_name
WHERE condition;
Syntax of HAVING clause;
SELECT column_name(s)
FROM table_name
WHERE condition
GROUP BY column_name(s)
HAVING condition
ORDER BY column_name(s);

The query stated above is incorrect as we cannot use the alias name while filtering data using the WHERE clause. It will throw an error.

The Union operator combines the output of two or more SELECT statements.
Syntax:
SELECT column_name(s) FROM table1
UNION
SELECT column_name(s) FROM table2;
Let’s consider the following example, where there are two tables - Region 1 and Region 2.

To get the unique records, we use Union.

The Intersect operator returns the common records that are the results of 2 or more SELECT statements.
Syntax:
SELECT column_name(s) FROM table1
INTERSECT
SELECT column_name(s) FROM table2;

The Except operator returns the uncommon records that are the results of 2 or more SELECT statements.
Syntax:
SELECT column_name(s) FROM table1
EXCEPT
SELECT column_name(s) FROM table2;

Below is the SQL query to return uncommon records from region 1.
A Subquery in SQL is a query within another query. It is also known as a nested query or an inner query. Subqueries are used to enhance the data to be queried by the main query.
It is of two types - Correlated and Non-Correlated Query.
Below is an example of a subquery that returns the name, email id, and phone number of an employee from Texas city.
SELECT name, email, phone
FROM employee
WHERE emp_id IN (
SELECT emp_id
FROM employee
WHERE city = 'Texas');

Fig: Product Price table

select top 4 * from product_price order by mkt_price desc;

Now, select the top one from the above result that is in ascending order of mkt_price.

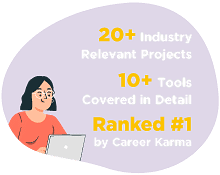

The SQL query is as follows:

The output of the query is as follows:


Fig: Products table

Fig: Sales order detail table
We can use an inner join to get records from both the tables. We’ll join the tables based on a common key column, i.e., ProductID.

The result of the SQL query is shown below.

You must be prepared for this question thoroughly before your next data analyst interview. The stored procedure is an SQL script that is used to run a task several times.
Let’s look at an example to create a stored procedure to find the sum of the first N natural numbers' squares.

Output: Display the sum of the square for the first four natural numbers


Here is the output to print all even numbers between 30 and 45.


Data joining can only be carried out when the data comes from the same source.
Data blending is used when the data is from two or more different sources.
E.g: Combining two or more worksheets from the same Excel file or two tables from the same databases.
All the combined sheets or tables contain a common set of dimensions and measures.
E.g: Combining the Oracle table with SQL Server, or combining Excel sheet and Oracle table or two sheets from Excel.
Meanwhile, in data blending, each data source contains its own set of dimensions and measures.
LOD in Tableau stands for Level of Detail. It is an expression that is used to execute complex queries involving many dimensions at the data sourcing level. Using LOD expression, you can find duplicate values, synchronize chart axes and create bins on aggregated data.
Feature selection is the process of selecting a subset of relevant features from a larger set of variables or predictors in a dataset. It aims to improve model performance, reduce overfitting, enhance interpretability, and optimize computational efficiency. Here's an overview of the process and its importance:
Importance of Feature Selection:
- Improved Model Performance: By selecting the most relevant features, the model can focus on the most informative variables, leading to better predictive accuracy and generalization.
- Overfitting Prevention: Including irrelevant or redundant features can lead to overfitting, where the model learns noise or specific patterns in the training data that do not generalize well to new data. Feature selection mitigates this risk.
- Interpretability and Insights: A smaller set of selected features makes it easier to interpret and understand the model's results, facilitating insights and actionable conclusions.
- Computational Efficiency: Working with a reduced set of features can significantly improve computational efficiency, especially when dealing with large datasets.
There are mainly 2 types of connections available in Tableau.
Extract: Extract is an image of the data that will be extracted from the data source and placed into the Tableau repository. This image(snapshot) can be refreshed periodically, fully, or incrementally.
Live: The live connection makes a direct connection to the data source. The data will be fetched straight from tables. So, data is always up to date and consistent.
Joins in Tableau work similarly to the SQL join statement. Below are the types of joins that Tableau supports:
A Gantt chart in Tableau depicts the progress of value over the period, i.e., it shows the duration of events. It consists of bars along with the time axis. The Gantt chart is mostly used as a project management tool where each bar is a measure of a task in the project.










From the above map, it is clear that states like Washington, California, and New York have the highest sales and profits. While Texas, Pennsylvania, and Ohio have good amounts of sales but the least profits.


Treemaps are used to display data in nested rectangles.
Heat maps can visualize measures against dimensions with the help of colors and size to differentiate one or more dimensions and up to two measures.
You use dimensions to define the structure of the treemap, and measures to define the size or color of the individual rectangles.
The layout is like a text table with variations in values encoded as colors.
Treemaps are a relatively simple data visualization that can provide insight in a visually attractive format.
In the heatmap, you can quickly see a wide array of information.








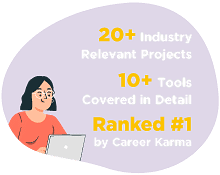
There are two ways to create a Pandas data frame.


To create a DataFrame in Python, you need to import the Pandas library and use the read_csv function to load the .csv file. Give the right location where the file name and its extension follow the dataset.

To display the head of the dataset, use the head() function.

The ‘describe’ method is used to return the summary statistics in Python.


You can use the column names to extract the desired columns.

num = np.array([[1,2,3],[4,5,6],[7,8,9]]). Extract the value 8 using 2D indexing.

Since the value eight is present in the 2nd row of the 1st column, we use the same index positions and pass it to the array.


Since we only want the odd number from 0 to 9, you can perform the modulus operation and check if the remainder is equal to 1.

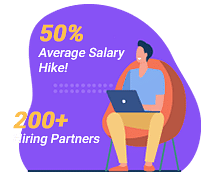

You can either use the concatenate() or the hstack() function to stack the arrays.

Suppose there is an emp data frame that has information about a few employees. Let’s add an Address column to that data frame.

Declare a list of values that will be converted into an address column.

To generate Random numbers using NumPy, we use the random.randint() function.


To find the unique values and number of unique elements, use the unique() and nunique() function.

Now, subset the data for Age<35 and Height>6.


Below is the result sine graph.





So, those were the 65+ data analyst interview questions that can help you crack your next data analyst interview and help you become a data analyst.
Now that you know the different data analyst interview questions that can be asked in an interview, it is easier for you to crack for your coming interviews. Here, you looked at various data analyst interview questions based on the difficulty levels. And we hope this article on data analyst interview questions is useful to you.
On the other hand, if you wish to add another star to your resume before you step into your next data analyst interview, enroll in Simplilearn’s Data Analyst Master’s program, and master data analytics like a pro!
Unleash your potential with Simplilearn's Data Analytics Bootcamp. Master essential skills, tackle real-world projects, and thrive in the world of Data Analytics. Enroll now for a data-driven career transformation!
To prepare for a data analyst interview, review key concepts like statistics, data analysis methods, SQL, and Excel. Practice with real datasets and data visualization tools. Be ready to discuss your experiences and how you approach problem-solving. Stay updated on industry trends and emerging tools to demonstrate your enthusiasm for the role.
Data analyst interviews often include questions about handling missing data, challenges faced during previous projects, and data visualization tool proficiency. You might also be asked about analyzing A/B test results, creating data reports, and effectively collaborating with non-technical team members.
An example to answer this question would be - “When considering me for the data analyst position, you'll find a well-rounded candidate with a strong analytical acumen and technical expertise in SQL, Excel, and Python. My domain knowledge in [industry/sector] allows me to derive valuable insights to support informed business decisions. As a problem-solver and effective communicator, I can convey complex technical findings to non-technical stakeholders, promoting a deeper understanding of data-driven insights. Moreover, I thrive in collaborative environments, working seamlessly within teams to achieve shared objectives. Hiring me would bring a dedicated data analyst who is poised to make a positive impact on your organization."
Yes, data analyst interviews often include a coding component. You may be asked to demonstrate your coding skills in SQL or Python to manipulate and analyze data effectively. Preparing for coding exercises and practicing data-related challenges will help you succeed in this part of the interview.
The level of stress in a data analyst role can vary depending on factors such as company culture, project workload, and deadlines. While it can be demanding at times, many find the job rewarding as they contribute to data-driven decision-making and problem-solving. Effective time management, organization, and teamwork can help manage stress, fostering a healthier work-life balance.
Shruti is an engineer and a technophile. She works on several trending technologies. Her hobbies include reading, dancing and learning new languages. Currently, she is learning the Japanese language.
How to Become a Data Analyst: A Step-by-Step Guide
Data Analyst Resume Guide
Data Analyst vs. Business Analyst: A Comprehensive Exploration
Unleash Your 2024 Data Analytics Career with IIT Kanpur
Data Analyst vs. Data Scientist: The Ultimate Comparison
Business Intelligence Career Guide: Your Complete Guide to Becoming a Business Analyst
© 2009 -2024- Simplilearn Solutions
Follow us!
Company
Work with us
Discover
For Businesses
Learn On the Go!
Trending Post Graduate Programs
Trending Bootcamp Programs
Trending Master Programs
Trending Courses
Trending Categories
Trending Resources
source